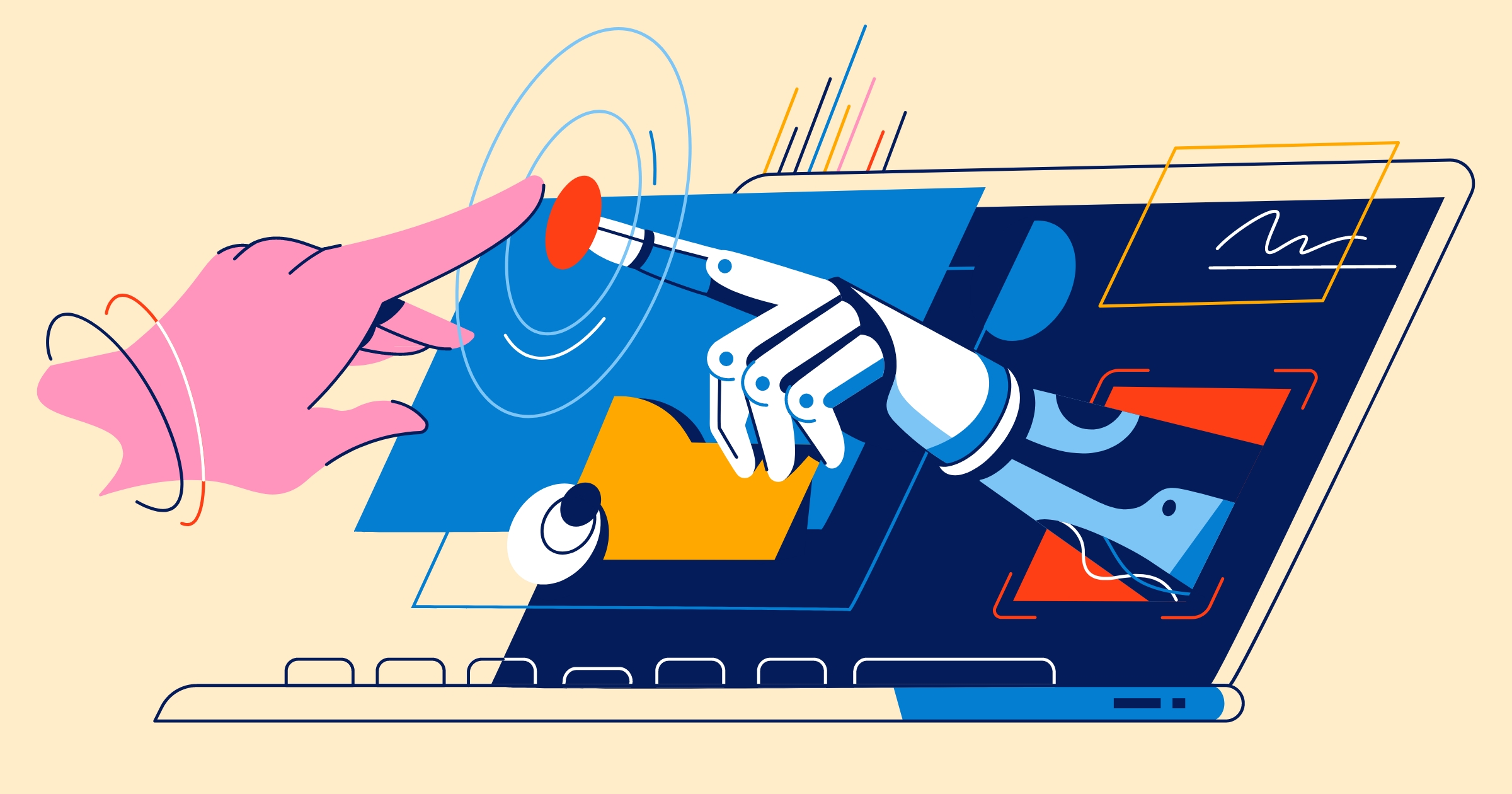
Artificial intelligence (AI) is more than a hot topic these days. It can be found everywhere, from ChatGPT to artificial customer service agents (chatbots), supply chain planning, evaluating medical treatment options, and even generating creative content such as online blogs, music, art, and images.
Across the world, organizations are using AI to improve decision-making, customer service, and personalization. AI provides insights, identifies opportunities, and inspires new products and services. Still, as incredible as AI can be, its rapid integration into every aspect of our lives poses some significant and ever-evolving risks.
As organizations continue to rely on outsourced products and services, the proliferation of AI represents yet another emerging risk domain that third-party risk managers must be able to identify, assess, manage, and mitigate, but this might be easier said than done. After all, AI is a broad term and covers a wide range of technology, use cases, and applications.
We’ve created this AI primer to help third-party risk managers gain a fundamental understanding of artificial intelligence to better understand some of the AI products, services, and risks that must be considered in their third-party risk management (TPRM) practices.
What Is Artificial Intelligence?
Artificial intelligence, referred to as AI, is a subset of computer science that aims to create systems capable of performing tasks that require human intelligence. These tasks include image and speech recognition, decision-making, and language translation. While both AI and automation involve machines performing tasks previously done by humans, they're not the same. Automation involves machines following pre-programmed rules, while AI involves machines that can learn and adapt from data.
The Two Types of AI
There are two primary types of AI to know. These are narrow AI, which is programmed to perform a specific task (like making music recommendations on Spotify), and general AI, which is a system with generalized human cognitive abilities, that can understand, learn, adapt, and implement knowledge in a broad range of tasks. Let’s discuss further.
- Narrow AI (or weak AI): Narrow AI refers to AI systems that are designed to perform a specific task. This could include things like voice recognition (like Siri or Alexa), recommendation systems (like Netflix or Amazon), or image recognition systems (like Face ID or Google Lens). Narrow AI operates under a limited set of constraints and contexts, and it doesn't possess the ability to understand, learn, or apply knowledge beyond its specific function.
Although the term "weak" is used, it doesn't mean that these systems are inferior. It merely refers to the scope of tasks that these systems can perform. In fact, narrow AI is quite powerful and forms the backbone of many AI applications we use daily. - General AI (or strong AI): General AI refers to a type of AI that can understand, learn, adapt, and implement knowledge across a broad range of tasks at a level equal to that of a human being. Unlike narrow AI, which is designed for specific tasks, general AI can transfer knowledge from one domain to another and make decisions in unfamiliar situations.
Currently, general AI is largely a theoretical concept without any existing examples. The development of general AI would represent a significant advancement in its field, as it would mean creating machines with the ability to perform any intellectual task that a human being can do.
AI Concepts and Terms to Know
Now that you understand the difference between narrow AI and general AI, learning some additional AI concepts and applications is helpful:
- Machine Learning (ML): This is a technique used to build and train AI models. Instead of being explicitly programmed, these models are trained on a large amount of data, learning to recognize patterns and make predictions based on these patterns.
- Artificial Neural Networks (ANNs): Draws inspiration from the intricate neural networks found in the human brain. These computational systems can learn and gradually enhance their performance, enabling them to identify patterns and make informed decisions.
- Deep Learning: A subset of ML that uses neural networks with many layers (hence "deep"). This technique is particularly effective for complex image and speech recognition tasks.
- Natural Language Processing (NLP): This teaches AI systems to understand, interpret, and generate human languages. This technology is behind chatbots and voice assistants like Siri or Alexa.
- Computer Vision: A field that focuses on enabling AI to interpret and understand visual data. It's used in applications like facial recognition systems, self-driving cars, and image editing software.
- Generative AI: This is a type of AI system that has become popular mostly due to systems like ChatGPT. In response to a prompt from a user, it can generate anything from text, images, voices, and other media. It uses ANNs to identify patterns and structures within existing data to generate new content.
Third-Party Risk Managers Should Understand How Industries Are Using AI
AI has permeated almost every industry, driving efficiency, accuracy, and innovation. As a third-party risk manager, understanding where and how AI is used in your industry can provide valuable context for assessing current and future vendor relationships and risks.
Here are some of the primary industries using AI and examples of how they are using it:
- Healthcare is increasingly leveraging AI to improve patient outcomes, reduce costs, and enhance operational efficiency:
- Disease diagnosis: AI algorithms can analyze medical images to detect diseases such as cancer at early stages.
- Personalized medicine: AI analyzes patient data to develop personalized treatment plans.
- Drug discovery: AI can streamline the drug discovery process, identifying potential compounds and predicting their effects more rapidly than traditional methods.
- Financial institutions use AI to enhance decision-making, improve customer service, and detect fraudulent activities:
- Risk assessment: AI models are used to predict risk and inform lending decisions.
- Fraud detection: AI systems can analyze transaction patterns to identify potentially fraudulent activity.
- Customer service: Many financial institutions use AI-powered chatbots for efficient customer service.
- Retail uses AI to enhance customer experience, streamline supply chain operations, and personalize marketing:
- Personalized shopping: AI algorithms analyze customer data to offer personalized product recommendations.
- Inventory management: AI is used to forecast demand and optimize inventory levels.
- Customer service: AI chatbots can handle a variety of customer inquiries, improving efficiency and customer satisfaction.
- Manufacturing production lines, quality assurance, and predictive maintenance are being revolutionized by AI:
- Quality control: AI systems can analyze images from production lines in real-time to detect and rectify defects.
- Predictive maintenance: AI can predict equipment failures, reducing downtime and maintenance costs.
- Supply chain optimization: AI predicts demand and optimizes logistics, improving efficiency and reducing costs.
- In agriculture, AI is becoming increasingly crucial, enhancing yield, optimizing resources, and improving sustainability:
- Precision farming: AI systems analyze data from satellites and sensors to provide actionable insights on crop health, watering needs, and pest/disease detection.
- Automated machinery: AI-powered drones and autonomous tractors can perform tasks such as seeding, spraying, and harvesting.
- In education, AI is transforming the industry, personalizing learning experiences and automating administrative tasks:
- Personalized learning: AI systems analyze individual learning patterns and adapt content to enhance learning outcomes.
- Automation of administrative tasks: AI can automate tasks such as grading and scheduling, allowing educators to spend more time on teaching.
The Leading Organizational and Third-Party Risks Present When Using AI
With the growth of AI also comes risks that can pose danger to your organization, especially with so many types of AI and different use cases. Maybe you’ve been asked to perform due diligence on an AI vendor, or one of your current vendors is using AI to handle some of its data.
Before your organization uses AI, or contracts with a third party to provide AI-driven products or services, you should be aware of the risks involved:
- Data privacy risks: AI systems thrive on data, often of a personal or sensitive nature, but if you’re not aware of what data is being used for the AI system, it could put your organization at risk for cybersecurity incidents or regulatory fines, such as the following:
- Inadequate data protection measures may lead to data breaches, causing financial and reputational damage.
- AI may unintentionally infringe upon privacy laws if not designed and deployed with strict adherence to regulations, such as the EU’s General Data Protection Regulation. There’s also a number of state privacy laws organizations need to follow.
- Bias and fairness risks: AI systems can inadvertently perpetuate or amplify biases if trained on skewed data, such as:
- Decisions made by biased AI, ranging from hiring to loan approvals, can unjustly disadvantage certain groups, leading to potential legal and reputational repercussions.
- Bias in AI systems can undermine their effectiveness and user acceptance.
- Explicability and transparency risks: The decision-making process of advanced AI models, such as deep learning, can be unclear. The algorithms can be difficult to understand and explain to others. This could lead to:
- Users not understanding how an AI system arrives at a decision, resulting in mistrust and hindered adoption.
- Lack of explicability, which presents legal risks, especially in regulated industries where decisions must be auditable.
- Security risks: Like all digital systems, AI systems are susceptible to cyberattacks. Hackers are quick to learn the systems and how they can misuse them. It can lead to new types of malware and phishing attacks that are even more sophisticated. For example:
- Adversarial attackers can manipulate AI inputs to produce misleading results.
- A compromised AI system might be used maliciously to carry out attacks or leak information.
- Intellectual property risks: Issues involving intellectual property rights in AI are a significant concern, such as:
- Misappropriation of proprietary AI technologies which may occur without proper protections.
- IP risks may arise when determining the ownership of AI-generated content or inventions.
- Regulatory compliance risks: Compliance with existing and evolving AI laws and regulations is a complex challenge given the global nature of digital services. Inconsistency in how countries approach and seek to legislate AI rules and laws means complexity for organizations utilizing AI products or services. Already, the EU has passed the Artificial Intelligence Act, with sweeping regulations that govern how AI can be used. Consider the following:
- Discrepancies in AI rules across jurisdictions, especially concerning privacy and bias, can result in severe penalties if not adhered to.
- The landscape of AI regulations is changing rapidly, requiring constant vigilance and adaptability.
- Vendor business continuity risks: Relying on third-party AI vendors can pose risks if the vendor's operation faces disruptions. For example:
- The organization's operations can be disrupted if an AI vendor goes out of business, or their service is interrupted, especially if the organization relies on AI to perform critical business functions.
- Changes in a vendor's policies or ownership can also affect their AI products and services.
- Reputation damage: Poorly executed AI can negatively impact your organization's reputation and damage your brand. Scenarios like the following may occur:
- Inaccurate or ineffective automated customer service channels or agents can frustrate customers and decrease their satisfaction.
- Overdependence on AI solutions removes the "human factor" from customer interactions, often resulting in re-work, lower issue resolution rates, and distrust in the company.
As AI continues its forward march, industries are rapidly finding innovative applications to harness its power. Third-party risk managers and teams who understand the AI basics, its various applications, and the risks associated with its use are better positioned to begin integrating vendor-related AI risk identification, assessment, and management into their third-party risk management practices and frameworks.
Related Posts
How to Manage Evolving Third-Party AI Risks
Despite the prevalence of artificial intelligence (AI) in today’s world, it seems as though humans...
Mitigating AI Vendor Risk With Questionnaires
The use of artificial intelligence (AI) has exploded within a few short years, with no signs of...
Third-Party Data Protection: Are Your Vendors Prepared?
Cybersecurity incidents, such as data breaches and ransomware attacks, have become increasingly...
Subscribe to Venminder
Get expert insights straight to your inbox.
Ready to Get Started?
Schedule a personalized solution demonstration to see if Venminder is a fit for you.